|
Enhancing Graph Representation Learning Through Self-Supervision: An Augmentation Perspective | 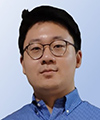 | BO Jianyuan PhD Candidate School of Computing and Information Systems Singapore Management University | Research Area Dissertation Committee Research Advisor Dissertation Committee Member |
| | Date 3 May 2024 (Friday) | Time 9:00am – 10:00am | Venue Meeting room 5.1, Level 5 School of Computing and Information Systems 1, Singapore Management University, 80 Stamford Road Singapore 178902 | Please register by 2 May 2024. We look forward to seeing you at this research seminar. 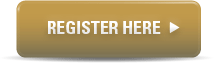
|
|
|
| ABOUT THE TALK Graph representation learning is pivotal for analyzing graph-structured data across diverse domains, yet traditional supervised methods are hindered by data scarcity and the extensive efforts required for labeling. Graph Self-supervised Learning (SSL) emerges as an effective alternative by leveraging inherent graph structures without explicit labels. It can be categorized into two main types: contrastive methods, which emphasize learning through differentiation, and generative methods, which focus on reconstruction. Together, these methods offer a comprehensive framework for enhancing SSL approaches in graph learning. However, each type also presents its own unique challenges and limitations.
To address these limitations, this dissertation proposal delves into Graph SSL from an augmentation perspective with two innovative aspects (1) adaptive augmentation sampling for contrastive learning, as demonstrated by our proposed Graph-centric Contrastive framework for Graph Matching (GCGM), utilizes a diverse pool of augmentations to enhance unsupervised graph matching without the need of any side information, and (2) the unification of contrastive and generative pre-training strategies, through our novel Contrastive Masked Node Reconstruction (CORE) framework. This framework utilizes node masking as a special augmentation, combining the strengths of both paradigms to enhance performance in node and graph classification tasks. Our experimental results highlight the superior performance of our proposed framework compared to existing SSL methods across various datasets. | | ABOUT THE SPEAKER BO Jianyuan is a PhD candidate in Computer Science at the School of Computing and Information Systems at SMU, under the supervision of Assistant Professor Fang Yuan. His research primarily focuses on graph representation learning, unsupervised learning, and graph neural networks. |
|
|
|
|