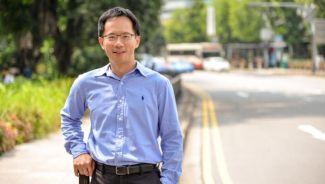
Assistant Professor Cheng Shih-Fen of the SMU School of Information Systems builds agent-based models to better understand complex systems and optimise their performances.
Photo Credit: Cyril Ng
By Grace Chua
SMU Office of Research (10 October 2014) – Experiments are the foundation of many scientific fields. For instance, in experimental physics or chemistry, experiments under controlled conditions are often needed to test hypotheses. But when it comes to management and social sciences, it is hard to conduct large-scale, real-life experiments.
To make better predictions, test hypotheses or generate new recommendations, models come in handy, especially the agent-based models that Assistant Professor Cheng Shih-Fen specialises in developing.
How to build an agent-based model
Traditionally, models are made in a top-down fashion, built on a set of equations that try to describe the workings of certain phenomena. However, this paradigm is not always possible, explains Professor Cheng of the Singapore Management University (SMU) School of Information Systems.
“So instead, we try to understand an individual agent’s behaviours,” he says. “Once we are certain that these behaviours are correct, we then try to put the agents together and see how they interact.” Often, complex interactions and patterns can emerge from simple rules about agents’ behaviour.
An agent can be anyone or anything, such as an individual, company or government, that is able to make independent choices, explains Professor Cheng. For example, agents might be ‘smart trading’ software programmes that are designed to execute certain types of trades automatically. Or they might be taxi drivers in a city who have to decide where and when to pick up customers. In an ecological model, agents might even be individual animals trying to survive and breed.
According to Professor Cheng, whatever the particular agent might be, there are two underlying principles to agent-based modelling. “Firstly, you do not get to control all the individuals. You can assume they are intelligent, or follow some kind of a behavioural model,” he explains.
“Secondly, you must have some way of defining how agents interact with each other. For example, in a financial market, your rules of engagement are basically the rules of the market, such as how orders are matched and how prices are set.”
When robots run the market
Professor Cheng, who is originally from Taiwan, did his doctoral work at the University of Michigan, Ann Arbor, in the Department of Industrial and Operations Engineering. At that time, the use of software to automate decision making processes like trading and supply chain operations was growing.
“We are in a world where a lot of important operations are being automated,” Professor Cheng says. “In these early phases, you are going to see hybrid systems where the majority of the agents are still human decision makers. I would like to understand the impact of introducing new technology to a particular area that is dominated by human beings.”
Agent-based models could also help understand the impact of financial regulation, or suggest what kind of regulation is needed, he says. One of his key research areas is in financial markets, especially commodity markets. “Imagine that the market is populated with automated trading programmes, with different banks and hedge funds all using trading programmes that look for similar market signals,” he elaborates.
Research presented by Professor Cheng and his colleagues in the 2010 issue of Review of Futures Markets showed that when such ‘smart trading’ programmes make up a certain proportion of the market, say greater than 60 percent, the market would start to become unstable and move in a more volatile fashion.
The Flash Crash on the afternoon of 6 May 2010 illustrated the dangers of an increasingly automated market. Within just 20 minutes, the Dow Jones Industrial Average plunged about 1,000 points, the biggest one-day point decline in Dow Jones Industrial Average history. Share prices of some heavily traded companies like Procter & Gamble and Accenture behaved oddly, dropping to as low as one cent or hitting $100,000.
High frequency trading programmes were fingered as a possible cause of the Flash Crash. This led Professor Cheng to think that most people have yet to fully understand the dynamics of automated programmes. “This demonstrates that the traditional way of modelling the market should really be completely re-thought. We should begin to explore the potential of using agent-based models as experimental platforms for both understanding market dynamics and designing regulations.”
Agent-based models for urban mobility
With urban mobility becoming a high priority in densely populated cities, Professor Cheng and his colleagues have decided to study taxi operations in Singapore. Using a large data set from a local taxi fleet to analyse the behaviour of cabbies, they showed that top earners anticipated where the customers would be, and moved elsewhere when they had too much competition in a given area.
With the help of an intelligent decision system based on both real-time demand and location information, drivers of all skill levels could benefit. Not only can they receive driving guidance and increase their revenue as a result of enhanced mobilisation, customers also get to enjoy prompt service.
In an upcoming project, Professor Cheng intends to find creative low-cost solutions to better manage both expected and unexpected traffic demand surges. For example, the Singapore government is encouraging the growth of the meetings, incentives, conferencing and exhibitions (MICE) industry. But events like the Singapore Grand Prix or large international conferences may generate concentrated human flows in certain areas. “If you have no space to expand your transportation infrastructure, you have to find ways to expand the productivity of your existing system,” he says.
Professor Cheng admits that the one challenge he faces is convincing his peers that agent-based modelling is a sound approach, as it is still a relatively new tool in many fields of management and social sciences. Many researchers are accustomed to a top-down approach, and in domains where good data sets are lacking, validating models can be difficult.
“I am not saying it is going to replace the traditional approach, but this paradigm could be a worthy alternative when other approaches fail to deliver satisfactory results,” Professor Cheng concludes.
Back to Research@SMU Issue 19 (Oct 2014)