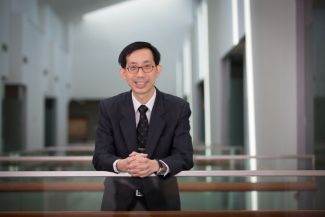
The wealth of content generated on social media platforms is a virtual gold mine for businesses looking to better engage their target audiences and enhance their marketing efforts.
Professor Lim Ee Peng, who is also the Director of Living Analytics Research Centre at SMU's School of Information Systems, has developed new analytics models and techniques for analysing different types of social media data that can be used for a variety of applications.
Specifically, the aim is to enable organisations to analyse small data (short messages), large data (collection of tweets from one user) or very large data (information generated by a community) and overcome the challenges associated with analysing these three types of data.
Using small data for geolocation
When it comes to small data, Prof Lim’s study tackles the problem of determining the location of social media users based on their social media activity. Performing such “fine-grained geolocation” is very challenging given the many possible locations of users.
However, being able to do so has many useful applications. For example, if it is possible to automatically determine that the post “Oh no, my bus has not shown up after 20 minutes’ wait” was posted at a bus stop outside Tanah Merah Ferry Terminal, the user would then be able to receive immediate suggestions for alternative transport options.
As a single social media post contains very little content, the research proposes using content from the other social media posts of the same user to enhance the accuracy of geolocation.
These include posts that are generated around the same time as the original one, which are likely to be from nearby locations, as well as posts that contain keywords possibly used at repeatedly visited venues. For example, it is quite common to find people mentioning “office” when they are at work places.
Using this technique, the target post can be more accurately matched with words that are likely to be found in other posts generated from the posting venues. This approach has been shown to improve accuracy over other advanced solutions by 15%, reveals Prof Lim.
Accurate profiling with large data
Social media content can also be analysed by aggregating all data generated by one user to create a profile for the purpose of personalising product recommendation, job search, and content suggestion applications.
Prof Lim’s study found that a significant proportion of users may disclose only selective information about themselves – a practice known as “selective self-disclosure” – by not generating any content or generating very little content, as well as by suppressing specific topics or opinions.
However, the research showed that an accurate profile of a user can still be generated by using information about the user's neighbours and behaviour on social media. “Organisations that wish to establish good relationships and communication platforms with audiences through social media have to realise that they should profile their audiences by not only focusing on what they say or disclose on social media, but also how they behave and who they choose to interact with to derive a more accurate judgement of consumer needs and preferences,” says Prof Lim.
Prof Lim’s research also developed a model for selecting suitable social media influencers for marketing campaigns.
This involves automatically discovering “topic-specific influencers” by determining which social media user accounts generate good content on a certain topic and attract followers and follower interaction on that topic.
Analysing large data without human labelled data
Analysing social media data at the community level within a single social media platform or across multiple platforms is known as analytics with very large data.
Unlike the cases of small and large data, however, the analysis of such data must take into account the possibility of users operating multiple accounts across different social media sites. Overcoming this “user identity linkage problem” will require matching user accounts from different social media platforms that belong to the same users.
Prof Lim’s research has developed solutions that do not require human experts to identify known accounts belonging to the same user, also known as “human labelled data”.
“By combining similarities between accounts measured by different account features such as bio description or user name, our proposed solution can achieve better accuracy than some state-of-the-art solutions that require human labelled data,” says Prof Lim.
He adds: “In the experiments, our proposed solution has also shown to correctly find for a given target user account on one social media platform, say, Facebook, the matching account from another social media platform, say, Twitter, more than half the time; an accuracy level sufficient for many real world applications.”
View the video on this topic below: