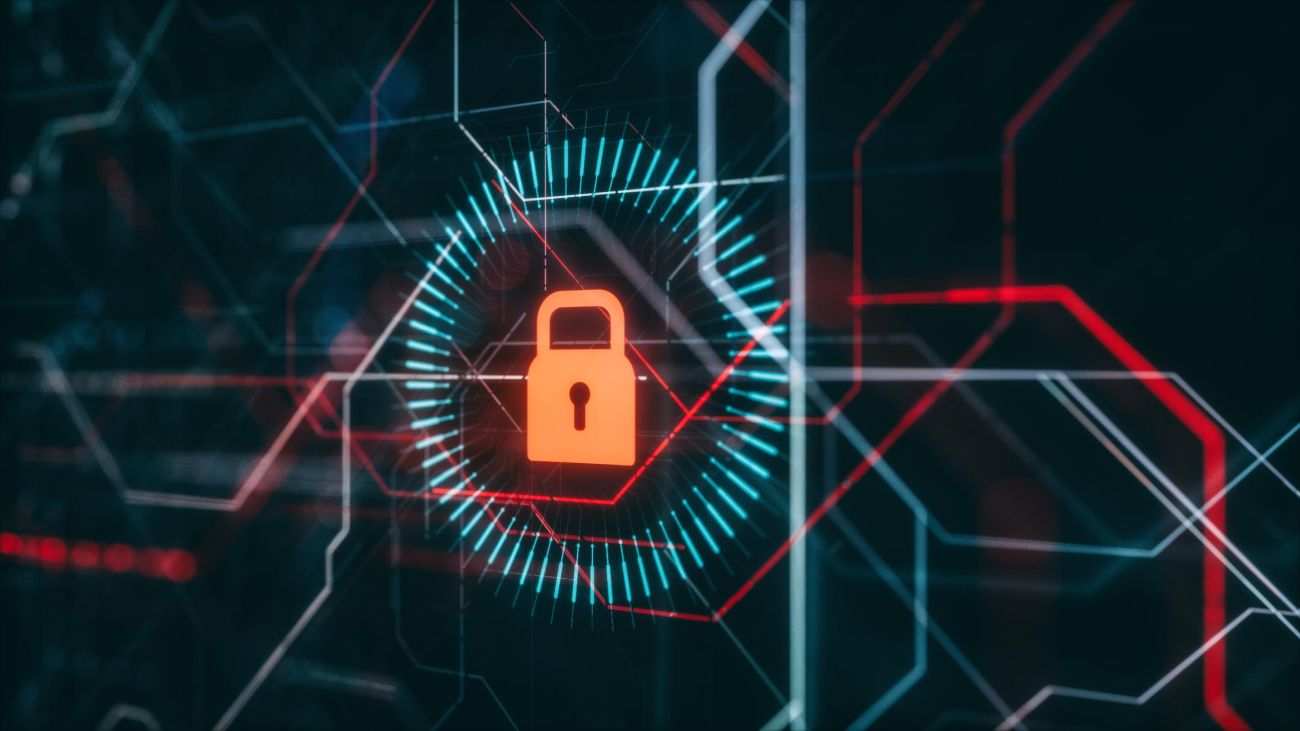
Cybersecurity is like a never-ending competitive ‘cat and mouse game’ between the legitimate protectors and the criminal attackers. Sometimes, the protectors become the attackers. What is clear though is that the entities that can move at greater speed and larger scale to make use of data to amplify their learning and intelligence will have the advantage, and ultimately prevail. Reflecting on the bewildering complexity, Steve Ledzian, Chief Technology Officer of Fire Eye Asia Pacific wondered: Could his company create a winning source of tools and models to keep up with—or better yet—keep ahead of the attacking opponent every time? Could an all-automated, AI machine-driven approach be the right way forward? Or was its existing approach, a symbiosis of human capability and AI-enabled machine support, good enough?
Established in 2004, FireEye was a publicly traded cybersecurity company headquartered in California with offices across North America, Europe, Asia Pacific, Middle East and Africa. The company specialised in providing software, hardware and support services in the cybersecurity field. FireEye had organised its cybersecurity solutions in a hub-and-spoke model designed to integrate machine-generated threat data from its detection and prevention products with its analytics, response expertise and orchestration technologies, delivered through a cloud-based cybersecurity operations platform. Armed with a moderately-sized team, the company had been able to drive its revenue from US$11.8 million in 2010 to US$889.2 million in 2019, relying on AI-based tools to execute tedious, repetitive tasks and a human + AI approach for tasks that involved decision making.
FireEye’s human + AI approach to cybersecurity had been helpful in several ways. Ledzian elaborated: “In cybersecurity, the biggest challenge is the skills gap. We do not have enough human analysts to do everything that we want to do. AI and machine learning (ML) tools help analysts take away some of those very tedious manual tasks that they are required to do, so that they are free to focus on higher-order tasks that require expert human decision-making skills.”
To guide its internal thinking on identifying scenarios in which a machine or a human expert would be the most effective approach to solve cybersecurity challenges, the firm had conceptualised an “automatability spectrum”, which took multiple factors into consideration to determine the degree of automatability of a task. It had implemented ML techniques to reduce the time to discover and distribute threat intelligence, as well as generate efficiencies across its product and services offerings. It had also applied AI solutions to baseline ‘normal’ behaviour to create alerts when anomalies and deviations occurred. However, implementing such solutions required benchmarking and validation of the solutions, and in turn, refining and training of the algorithms based on the findings. It also needed a mind-set shift in which analysts could start trusting a model and use a model’s findings in their analysis. Besides, AI solutions involved an iterative testing and retraining process, and benefits of its implementation were sometimes not immediately visible.
Given the long gestation period of AI solutions, could FireEye deliver its expertise seamlessly with the help of AI tools, arming human experts with the exact information they needed, when they needed it most. Could FireEye develop predictive AI tools that would foretell what a threat entity would do in the future?
Set in July 2020, the case explores how one of the world’s most renowned cybersecurity firms, FireEye, implemented AI-based solutions within the organisation to provide improved cybersecurity services to its clients. The case delves into the various strategies implemented by the firm for AI adoption using a specific example tool, and the continuing challenges faced by the firm amidst rising cyber threats. It is written by Steven Miller, Professor Emeritus of Information Systems, Singapore Management University (SMU) and Lipika Bhattacharya of the Centre for Management Practice (CMP) at SMU.
To read the case in full, please visit the CMP website by clicking here.