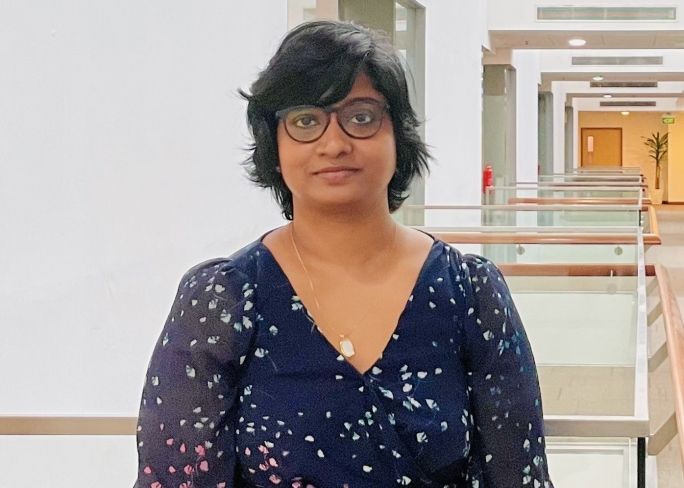
SMU Office of Research & Tech Transfer – Whether it is via personal diaries or family photo albums, the desire to keep a record of key moments in our lives seems part of human nature.
Now, courtesy of the internet, what was once a mostly private pastime enjoyed among family and friends can be publicly accessible.
Smartphones, personal computers and online storage providers have enabled exponential growth in the capacity to document our day-to-day lives – along with a proliferation of blogs and websites that share these life journeys.
It is known as life-logging, and with the advent of wearable cameras to continually record images of a person’s life from their point-of-view.
But what if we share too much? This is a particular issue when life-logging is used in hospitals as a memory support for elderly patients.
“Unbeknownst to the wearer, the wearable cameras automatically capture various sensitive information, such as bystanders, private situations and screens of computing devices such as laptops and mobile phones,” says Thivya Kandappu, an Assistant Professor of Computer Science at Singapore Management University (SMU).
“Hence, implicitly and unexpectedly, the images reveal more sensitive information, such as social connections. We believe life-log images are particularly vulnerable to this [privacy] threat due to their enhanced contextual and temporal continuity.”
Reminiscence-based therapy
Professor Kandappu and her co-researchers have examined the use of life-logging in a public health context where it is applied to enhance the quality of life for older adults.
“Ageing imposes major difficulties in older adults in memory performance as the ability to generate new synapses between neurons in response to external stimuli declines due to the deterioration of the brain function,” Professor Kandappu says.
“Visual life-logging (captured using wearable cameras of small form-factor) enables automatic and convenient recording of images of a person’s life from an egocentric point-of-view. This can subsequently be presented as a digital diary/storyboard – a collection of chronologically ordered images – to the older adults who can then retrieve the images and reminisce relevant episodic memories.
“Hence, reminiscence-based therapy is widely adopted to stimulate the memory recall process,” she adds.
But images captured by the cameras and uploaded to a server can be hacked and the stolen information misused. Image recognition technologies can find links to other people, scenes and objects to glean social affiliation networks, or enable social ties with other life-loggers to be inferred by observing co-located episodes with bystanders in the images.
The researchers find that an adversary can accurately infer the social relationships between life-loggers, by longitudinally observing their image feed, with 82 percent precision and 68 percent recall.
Professor Kandappu notes an “alarming rate of healthcare related data breaches recorded in the recent past”, such as the attack earlier this year on US medical services provider Shields Healthcare which exposed personal records of nearly two million people.
More recently in Australia, health insurer Medibank revealed that its four million customers have had their data exposed to a hacker.
“Data breaches are not uncommon and are usually not easy to foresee and prevent,” Professor Kandappu says. “It may not necessarily be the cloud server who is interested in the data – an adversary can unethically obtain the access.”
Selective obfuscation
To address the privacy threat due to inference of the life-logger’s social ties with bystanders and fellow life-loggers, Professor Kandappu and her colleagues have developed PrivacyPrimer, an automated platform.
“PrivacyPrimer is a user client application that acts as an intermediary – it periodically downloads the images from the wearable cameras and opportunistically obfuscates them before uploading to the host server,” she says.
The obfuscation uses commonly available image filters such as blurring, pixelating and masking. And with advances in computer vision and deep learning in regard to detecting and recognising faces, objects and meaningful text, privacy sensitive artefacts in photos can be removed.
The study began by examining life-log images obtained from 54 older adults to identify the artefacts, or visual cues, along with the context of the image that influenced an older life-logger’s ability to recall the events associated with the images.
Using the set of stimuli extracted, the researchers then proposed a set of obfuscation strategies that naturally balanced the trade-off between reminiscability and privacy while selectively obfuscating parts of the images.
“Using PrivacyPrimer, we have learned that such an optimal trade-off is not one-shot. It should be learned continuously with each new piece of information being revealed,” Professor Kandappu says.
“To be more specific, the trade-off implies temporal continuity, and it varies based on the visual cues captured in the current image, and the historical information that has been shared with the host server.”
Sensitivity of visual cues
The presence of a human face in a life-log image elicits better cue-based augmented memory recall – 31 percent higher in a reminiscability score compared with images with no human cue.
And the uniqueness of the context plays an important role in memory recall: on average, personal and unique locations prompt better memory recall – more than 28% improvement in reminiscability as opposed to the other visual cues.
The importance of these two factors must surely make the obfuscation choices more complicated.
“Indeed, while the visual cues act as proxies to stimulate reminiscence, exposing them imposes greater privacy risks,” Professor Kandappu says.
“In particular, we focus on a set of visual cues such as human faces and context details, and the developed algorithm chooses to obfuscate [them] based on the sensitivity of each of such cues.
“For example, through experimental studies, we learned that the users are able to recall their memory even when the face is obfuscated. They relied on other cues such as the shirt the bystander was wearing or salient objects that appeared in the image.”
The researchers' empirical findings show that the PrivacyPrimer platform yields a privacy-utility trade-off that only compromises, on average, a modest 13.4 percent on reminiscability scores while significantly improving privacy guarantees by around 40 percent.
And will this study lead to further research?
“As a continuation of memory enhancing therapeutic intervention work, I’m currently focusing on designing and developing wearable devices that can detect various cognitive features. For example, cognitive and affective states such as confusion and boredom,” Professor Kandappu says.
Back to Research@SMU November 2022 Issue