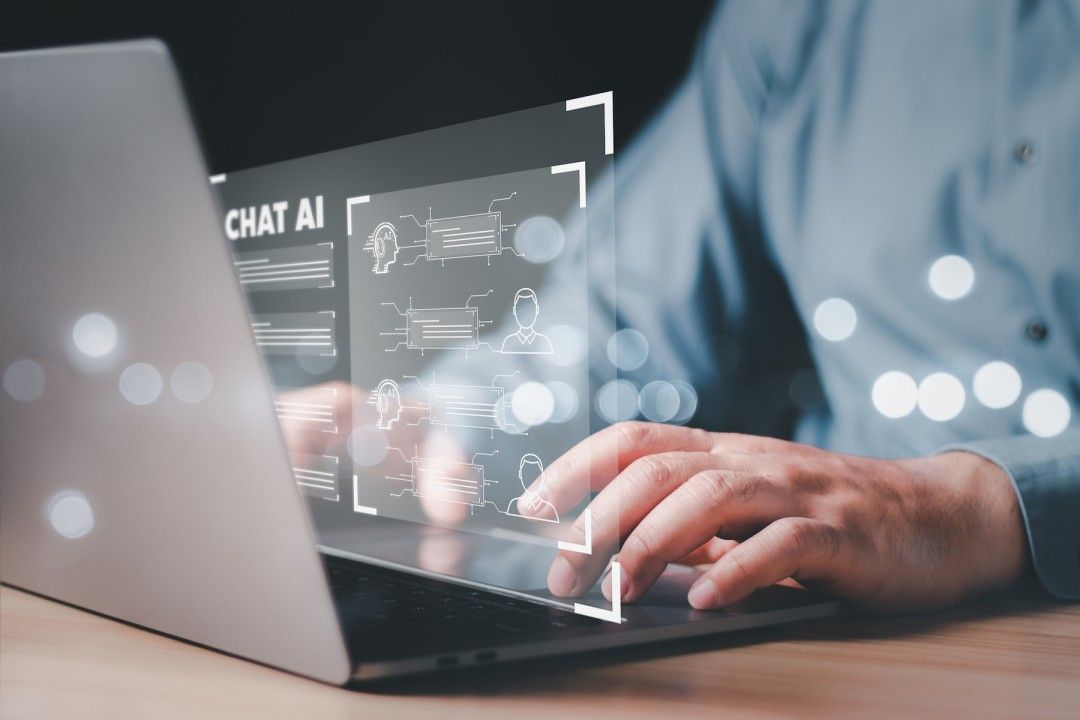
SMU Associate Professor Ouh Eng Lieh is developing Singapore’s first ChatGPT-based Prompt Tutor to provide real-time feedback to enhance student learning.
By Jovina Ang
SMU Office of Research – “Giving students immediate and frequent feedback makes online learning more effective,” Associate Professor Ouh Eng Lieh told the Office of Research.
However, based on how most online lessons are designed, questions could not be answered nor doubts clarified until students meet their instructor in the following face-to-face class.
The time delay of a few days to a few weeks can impede student learning as it might make it difficult for students to catch up and understand the subsequent topics in the course.
Learning also does not occur until the knowledge gained is stored in long‐term memory.
The Cognitive Load Theory (CLT) states that for short-term knowledge to be committed to long-term memory, there must be effective cognitive load management, repeated reinforcements and clarification of doubts.
“That’s why providing timely feedback is vital for maintaining a seamless and effective student learning experience. It also helps the student to manage the cognitive load and learn,” Professor Ouh explained to the Office of Research.
He added: “It has been found that reflection prompts, which are essentially reminders to get students to reflect and journal their learnings, can significantly improve learning by getting students to retain and apply what they have learned. Additionally, by leveraging ChatGPT, we can evaluate the degree of understanding by comparing what the student has written to the context or the topic of the lesson.”
“With these in mind, we wanted to develop a learning tool that can provide personalised prompts so that students could either have their questions answered or doubts clarified as they go through their online lesson. This tool, which we have fondly named ‘Prompt Tutor’, will also be programmed to assign additional exercises or generate a quiz for students to hone their learning,” Professor Ouh elaborated.
“And to the best of our knowledge, we believe that this will be the very first Prompt Tutor to be developed in Singapore for teaching computing courses in tertiary institutions,” Professor Ouh went on.
The research
Professor Ouh’s project is funded by an MOE Tertiary Education Research Fund (TRF) grant. An expected three years is needed to develop a fully functioning Prompt Tutor and conduct the experimental research.
He is collaborating with Associate Professor Tan Kar Way and Assistant Professor Lo Siaw Ling, both of whom are from Singapore Management University (SMU), as well as Dr. Lin Feng from Singapore University of Social Sciences (SUSS).
The research builds on Professor Ouh’s previous work where he has successfully developed a Doubt Identification Machine Learning Model to single out doubts from written reflections.
The integration of Prompt Tutor to SMU’s ITSS (Interactive Tutorial Software System) makes it possible for the research team to engineer real time feedback by providing the context to the machine learning algorithms.
The initial part of the research project entails developing a sufficiently accurate Prompt Tutor – essentially a Prompt Tutor that can provide at least 90 percent accurate responses to the student queries or detect doubts in the written reflections.
A total of 80 SMU students enrolled in introductory programming courses will be recruited to participate in the research project. 40 students will be exposed to the Prompt Tutor while the remainder 40 will not be.
There are four steps in the research methodology.
Step 1: Students will be required to watch a five-minute video and write a reflection on what they have learnt.
Step 2: With the use of the Doubt Identification Machine Learning Model, the Prompt Tutor will be programmed to detect doubts or inaccuracies in the student reflections.
Step 3: The Prompt Tutor will then evaluate the students’ written work by checking against the context and accuracy of the topic of the lesson. If any doubt or inaccuracy is detected, the Prompt Tutor will notify the student by sending a personalised prompt such as asking the student a follow-up question for him/her to further reflect and learn.
Step 4: If no inaccuracy is detected, the Prompt Tutor will either prompt the student with more exercises or generate a quiz to test understanding.
Implications of the research
This research has wide ranging applications beyond the teaching of Computer Science or Information Systems courses.
Given that the development of the Prompt Tutor is based on CLT, and therefore, discipline agnostic, its use can be easily extended to the teaching in fields such as Social Science, Medicine, and even languages.
Other than benefiting the students, the Prompt Tutor can benefit instructors – by reducing the time spent on student consultation.
Back to Research@SMU May 2024 Issue