|
|
Learning Dynamic Multimodal Networks
|
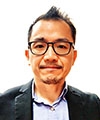
|
ANG Meng Kiat Gary
PhD Candidate
School of Computing and Information Systems
Singapore Management University
|
Research Area
Dissertation Committee
Research Advisor
Committee Members
External Member
|
|
|
Date
22 May 2023 (Monday)
|
Time
10:00am - 11:00am
|
Venue
Meeting room 5.1, Level 5.
School of Computing and Information Systems 1,
Singapore Management University,
80 Stamford Road
Singapore 178902
|
We look forward to seeing you at this research seminar.
Please register by 21 May 2023.
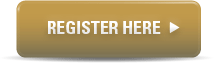
|
|
|
|
About The Talk
In this dissertation, we focus on modeling an important class of networks present in many real-world domains. These networks involve i) attributes of different modalities, also known as multimodal attributes; ii) multimodal attributes that are dynamic captured in the form of time-series, and/or iii) dynamic relationships that evolve with time. We refer to such networks as dynamic multimodal networks.
An example of static networks involving static multimodal attributes are networks of design objects in user interfaces (UI) formed from links between UI screens and their constituent UI elements, where the design objects may be associated with multimodal attributes such as visual UI screen and element images, textual UI descriptions and code. An example of dynamic networks involving dynamic multimodal attributes are networks between companies based on dynamic commercial relationships between companies which are further associated with dynamic multimodal attributes such as time-series of numerical stock prices, time-series of textual news, and time-series of categorical event attributes.
Despite the importance of modeling multimodality and dynamicity in networks, few existing works have addressed the challenges of modeling the diverse characteristics of multimodal network attributes and non-stationarity of dynamic networks and attributes. In this dissertation, we present a series of works that address such challenges of learning both static and dynamic multimodal networks. We also show that our proposed models out-perform state-of-the-art models on prediction and forecasting tasks on multiple real-world datasets.
|
|
Speaker Biography
Gary worked in the Monetary Authority of Singapore (MAS) before embarking on this PhD journey on a scholarship from MAS. He graduated from the University of Toronto with a bachelor’s degree in electrical engineering, and has master’s degrees from the National University of Singapore in knowledge engineering, as well as financial engineering. His research interests are in the areas of graph learning, time-series modeling, and applications of deep learning in finance and design. His research works have been accepted by conferences and journals such as the Annual Meeting of the Association for Computational Linguistics and ACM Transactions on the Web, and received an honorable mention award at the ACM Annual Conference on Intelligent User Interfaces in 2022. He received the SMU Presidential Doctoral Fellowship in 2022. He enjoys illustrating and generating art with algorithms in his spare time.
|
|