|
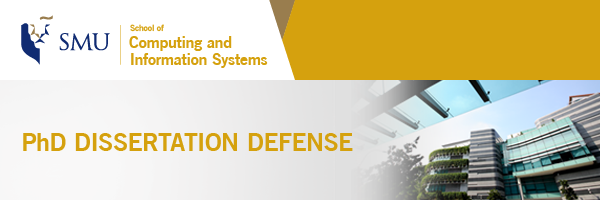 | | Incorporating Intrinsic Structures into Entity Matching and Representation Learning | 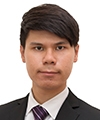 | LEE Ween Jiann PhD Candidate School of Computing and Information Systems Singapore Management University | Research Area Dissertation Committee Research Advisor Dissertation Committee Member External Member - BAO Zhifeng, Professor, School of Computing Technologies, STEM College, RMIT University
|
| | Date 25 June 2024 (Tuesday) | Time 3:00pm – 4:00pm | Venue Meeting room 4.4, Level 4 School of Computing and Information Systems 1, Singapore Management University, 80 Stamford Road Singapore 178902 | Please register by 24 June 2024. We look forward to seeing you at this research seminar. 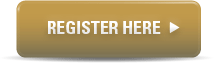
|
|
|
| ABOUT THE TALK In today's digital era, the proliferation of internet-connected devices and online services has generated vast amounts of user-generated content in various formats, such as text, visuals, and spatial information. Advanced deep learning techniques can extract valuable insights from this data, but challenges like fragmentation and a lack of cohesive structure persist to plague data quality. Addressing these challenges involves identifying and aggregating similar entities and obtaining representations that capture intrinsic properties of the data, especially for spatial context entities, often overlooked by traditional methods. Our research aims to enhance representation learning and data matching across graph, semi-ordered, and spatial data, improving applications like transportation, recommendation systems, and urban planning. This work is particularly relevant during the COVID-19 pandemic, where robust representation learning methodologies are required for contact tracing and risk profiling.
We introduce novel approaches to tackle the challenges of information amalgamation through entity matching and representation learning. In representation learning, our GeoNN model, detailed in "Latent Representation Learning for Geospatial Entities," captures spatially-aware embeddings using geodesic functions and multi-head attention. GeoNN's effectiveness is demonstrated across various contexts, offering an advancement in spatial representation learning and addressing gaps in current methodologies. Our entity matching method, outlined in "Robust BiPoly-Matching for Multi-Granular Entities" and "Robust Bidirectional Poly-Matching" challenges the assumption that entities must share similar granularity, allowing bidirectional one-to-many matching. This approach enhances robustness against noisy similarity values and diverse entity descriptions. Our work "Semi-Ordered Bidirectional Poly-Matching" addresses matching records within partially ordered datasets. In these papers, we offer proof of NP-hardness and introduce effective heuristics validated by large-scale experiments. | | ABOUT THE SPEAKER Ween Jiann Lee is a Ph.D. candidate at the School of Computing and Information Systems at Singapore Management University (SMU), where he is pursuing a doctoral degree in computer science. He holds a bachelor's degree in business management with a major in Operations Management and Analytics from SMU. His research interests focus on graph neural networks, representation learning, and matching problems, in which he has made significant contributions, showcasing his dedication to solving complex problems with practical applications. His work has been recognized at leading conferences and journals, including the IEEE ICDM, IEEE TKDE, and ACM TSAS. Aside from his academic pursuits, he is also an avid traveler, always eager to explore new places and cultures. He is committed to achieving a well-rounded balance between personal and professional growth. |
|