|
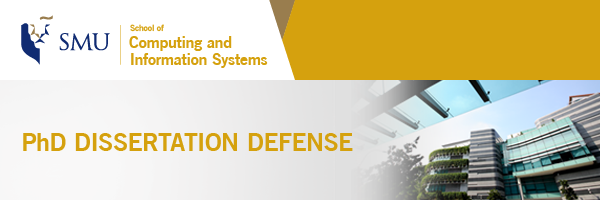 Disentangling User Preferences Towards Self-Interpretable Recommender Systems | 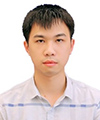 | TRAN Nhu Thuat PhD Candidate School of Computing and Information Systems Singapore Management University | Research Area Dissertation Committee Research Advisor Committee Members External Member - XU Guandong, Professor, School of Computer Science and Data Science Institute, University of Technology Sydney
|
| | Date 28 May 2025 (Wednesday) | Time 10:00am - 11:00am | Venue Meeting room 5.1, Level 5 School of Computing and Information Systems 1, Singapore Management University, 80 Stamford Road Singapore 178902 | Please register by 26 May 2025. We look forward to seeing you at this research seminar. 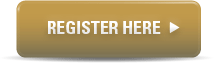
|
|
|
| ABOUT THE TALK Understanding user preferences remains a central challenge in recommender systems due to their inherently complex, unstructured, and multi-faceted nature, exacerbated by the sparsity of user interaction data. Traditional approaches often compress user interests into a single latent vector, overlooking the fact that user preferences are typically shaped by multiple underlying factors that differ across individuals. These latent drivers are not directly observable and must be discovered through unsupervised modeling, further complicated by limited historical interactions per user.
This thesis addresses these challenges by introducing a principled framework for multi-interest modeling, which disentangles user behaviors into multiple latent factors to better reflect the diversity of user preferences. The contributions are organized into two main directions: (i) modeling multiple user interests from interaction data through personalized clustering, multi-faceted grouping, and integrating interest sharing among users; and (ii) enhancing multi-interest user representations with side information, such as textual content, via cross-modal interest alignment. These innovations enable more accurate and granular user modeling, significantly improving recommendation performance across various real-world benchmarks.
A key novelty of this thesis lies in its systematic treatment of disentangled preference learning, where each model is designed to reveal interpretable and semantically meaningful user interest factors. This not only improves predictive performance but also supports model transparency: offering insights behind latent representations of user interest factors. Furthermore, the methods developed in this thesis contribute broadly to the fields of representation learning and interpretable AI, which could be applied for other kinds of sparse and heterogeneous data. | | SPEAKER BIOGRAPHY TRAN Nhu Thuat is a PhD candidate in Computer Science at Singapore Management University's School of Computing and Information Systems, supervised by Prof. Hady W. Lauw. His research explores multiple aspects of user preferences in recommendation systems, resulting in publications at major venues including KDD, ICLR, CIKM, WSDM, UAI, and TIST. He also serves as a program committee member for conferences such as KDD, ICLR, TheWebConf, WSDM, CIKM, and PAKDD, and as a reviewer for the journal IP&M. During his PhD, he has been awarded the SMU Presidential Doctoral Fellowship, the Singapore Data Science Consortium Fellowship, and the SCIS Dean's List. In his leisure time, he enjoys reading and outdoor sports. |
|