|
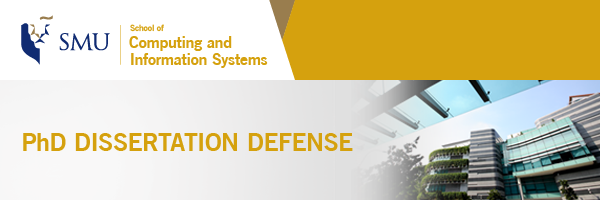 Boosting Symbolic Execution for Vulnerability Detection | 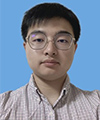 | TU Haoxin PhD Candidate School of Computing and Information Systems Singapore Management University | Research Area Dissertation Committee Research Advisor Co-Research Advisor Committee Members External Member - Marcel Böhme, Faculty Member, MPI SoftSec Research Group, Max Planck Institute for Security and Privacy, Germany
|
| | Date 9 May 2025 (Friday) | Time 3:00pm - 4:00pm | Venue Meeting room 5.1, Level 5 School of Computing and Information Systems 1, Singapore Management University, 80 Stamford Road Singapore 178902 | Please register by 8 May 2025. We look forward to seeing you at this research seminar. 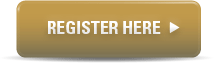
|
|
|
| ABOUT THE TALK Software systems written by humans tend to be unreliable and insecure, hence, vulnerabilities in them are inevitable. Symbolic execution has shown considerable potential in detecting diverse types of software bugs and also vulnerabilities that have severe security implications. However, existing symbolic execution engines still suffer from at least three fundamental limitations in memory modeling, path exploration, and structured input generation, which significantly impede existing engines for efficiently and effectively detecting software bugs and vulnerabilities.
The dissertation aims to boost existing symbolic execution engines by designing a new memory model, two new path exploration strategies, and a new test input generation solution to alleviate three key limitations to facilitate automatic vulnerability detection. Specifically, in the first work, we propose SymLoc, a vulnerability detection system that designs a new symbolic memory model. In the second work, we propose FastKLEE, a faster path exploration solution achieved by reducing redundant bound checking during execution. In the third work, we propose Vital to perform vulnerability-oriented path exploration for effective vulnerability detection. In the fourth work, we propose Cottontail, a LLM-driven concolic execution engine that could effectively generate highly structured test inputs for detecting vulnerabilities in parsing test programs.
The prototypes implemented in the dissertation are all open-sourced and have found more than 10 unknown vulnerabilities (e.g., buffer overflow and memory leakage) in widely used software systems, many of which have been assigned new CVEs (e.g., CVE-2024-55061). | | SPEAKER BIOGRAPHY TU Haoxin is currently a Dual-degree Ph.D. candidate at SMU (Singapore Management University) and DUT (Dalian University of Technology). He has finished and earned his first Ph.D. degree at DUT in December 2023. At SMU, he is supervised by Prof. Lingxiao JIANG and Prof. Xuhua DING. His research focuses on developing practical techniques and tools that can help improve the reliability and security of software systems (mainly system software such as compilers and Linux kernels). More information about Haoxin's research is available at https://haoxintu.github.io/. |
|