|
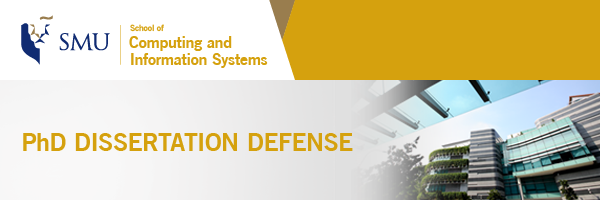 Cutting Through the Infodemic Efficiently: News Claims Surveillance and LLM-based Lightweight Fact Verification | 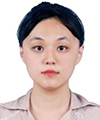 | ZHANG Xuan PhD Candidate School of Computing and Information Systems Singapore Management University | Research Area Dissertation Committee Research Advisor Committee Members External Member - Alton CHUA, Associate Professor, Wee Kim Wee School of Communication and Information, Nanyang Technological University
|
| | Date 2 June 2025 (Monday) | Time 1:00pm - 2:00pm | Venue Meeting room 5.1, Level 5 School of Computing and Information Systems 1, Singapore Management University, 80 Stamford Road Singapore 178902 | Please register by 29 May 2025. We look forward to seeing you at this research seminar. 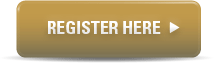
|
|
|
| ABOUT THE TALK Recently, the rise of deep learning technologies has offered the potential for accelerating the development of automated misinformation detection and verification. However, current technological capabilities and computational resources often prove inadequate for the exhaustive scrutiny required, rendering the enhancement of processing efficiency a critical imperative.
Given the vast amount of data on the internet, current technology and computational power often fall short in timely and accurate scrutiny of each piece of information, making the improvement of processing efficiency an urgent issue to address. Furthermore, effective fact-checking requires sophisticated reasoning capabilities. According to the characteristics of large language models (LLMs), only models of considerable size can emerge with advanced reasoning and understanding abilities. This demand places a higher challenge on computational resources. Moreover, even if these LLM theoretically support advanced fact-checking, efficiently adapting them for specific verification tasks remains a technical challenge. This thesis aims to improve automated misinformation monitoring and evidence-based fact verification systems by enhancing their efficiency and accuracy.
Accordingly, we address two primary challenges: In the first part, we address the challenge of more effective infodemic surveillance. We innovatively study rumor detection and virality prediction within a unified framework, as these are critical aspects of infodemic surveillance. The second part focuses on leveraging large language models (LLMs) for scalable fact verification. To harness LLMs without prohibitive expense, we develop an efficient pipeline. HiSS prompting breaks each claim into verifiable sub-claims; LLM-guided fine-grained feedback steers evidence retrieval; Chain-of-Preference Optimization aligns model reasoning with preferred multi-step thought chains; and hybrid LightTransfer models double inference speed with negligible accuracy loss. Together, these steps raise verification accuracy by up to 4.3 % while reducing compute requirements. | | SPEAKER BIOGRAPHY ZHANG Xuan is a Ph.D candidate in Computer Science at the SMU School of Computing and Information Systems, supervised by Professor GAO Wei. Her research interests stand in factuality-enhanced rumor claim surveillance and verification. |
|