|
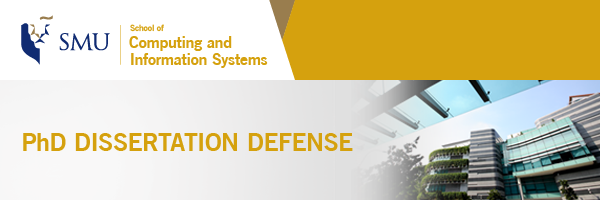 Towards Reliable ML: Data Attribution and Adversarial Robustness | 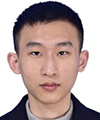 | ZHENG Xiaosen PhD Candidate School of Computing and Information Systems Singapore Management University | Research Area Dissertation Committee Research Advisor Committee Members External Member - Tianyu PANG, Senior Research Scientist, Sea AI Lab, Singapore
|
| | Date 23 May 2025 (Friday) | Time 2:00pm - 3:00pm | Venue Meeting room 5.1, Level 5 School of Computing and Information Systems 1, Singapore Management University, 80 Stamford Road Singapore 178902 | Please register by 21 May 2025. We look forward to seeing you at this research seminar. 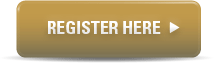
|
|
|
| ABOUT THE TALK Modern machine learning (ML) models achieve remarkable success, but face critical reliability challenges. This thesis advances two pillars of reliable ML systems: interpretability through data attribution and robustness against adversarial threats.
In the first part, we develop novel data attribution methods to elucidate the data-model relationship. We establish the critical role of memorization in model generalization through token-level influence analysis, extend sample-level attribution to diffusion models with effective approximation techniques, and introduce RegMix, a group-level approach that predicts data mixture performance using small-scale experiments. These contributions provide practitioners with scalable tools to audit training data impacts across modalities.
The second part exposes vulnerabilities in ML robustness through three adversarial perspectives. We reveal cascading failures in multi-agent LLM systems where single adversarial inputs propagate through million-agent networks, develop improved attacks achieving 99% success against state-of-the-art aligned models, and demonstrate how trivial "null models" exploit benchmark design flaws. Our findings challenge prevailing assumptions about LLM security and evaluation practices.
Collectively, this work bridges the gap between model capabilities and operational reliability. By advancing both explanatory frameworks for model decisions and exposing critical vulnerabilities, we provide insights for developing reliable ML systems that are both understandable and secure against emerging threats. | | SPEAKER BIOGRAPHY Xiaosen ZHENG is a fifth-year PhD Candidate in Computer Science at the SCIS, supervised by Prof. Jing JIANG. His research focuses on Data-Centric AI and AI Safety. |
|