|
Scalable Online Kernel Learning
Speaker (s):

LU Jing
PhD Candidate
School of Information Systems
Singapore Management University
|
Date:
Time:
Venue:
|
|
April 26, 2017, Wednesday
3:30pm - 4:30pm
Seminar Room 3.2, Level 3
School of Information Systems
Singapore Management University
80 Stamford Road
Singapore 178902
We look forward to seeing you at this research seminar.
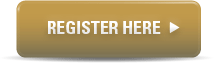
|
|
About the Talk
One critical deficiency of traditional online kernel learning methods is their increasing and unbounded number of support vectors (SV's), making them inefficient and non-scalable for large-scale applications. Recent studies on budget online learning have attempted to overcome this shortcoming by bounding the number of SV's. Despite extensive studied, budget algorithms usually suffer from sever drawbacks.
First, although existing algorithms attempt to bound the number of SV's at each iteration, most of them fail to bound the number of SV's for the averaged classifier, which is commonly used for online-to-batch conversation. To solve this problem, we propose a novel bounded online kernel learning method, Sparse Passive Aggressive learning (SPA), which is able to yield a final output kernel-based hypothesis with a bounded number of support vectors.
Second, existing budget learning algorithms are either too simple to achieve satisfactory approximation accuracy, or too computationally intensive to be scalable to large datasets. To overcome this limitation and make online kernel learning efficient and scalable, we explores two functional approximation based online kernel machine learning algorithms, Fourier Online Gradient Descent (FOGD) and Nystr\"{o}m Online Gradient Descent (NOGD).
We theoretically prove that the proposed algorithms achieve an optimal regret bound, and empirically show that the new algorithms outperform various bounded kernel-based online learning algorithms.
About the Speaker
LU Jing is a PhD student in the School of Information Systems (SIS), Singapore Management University (SMU), Singapore. Prior to joining SMU, She received her Bachelor’s Degree in Honor School, Harbin Institute of Technology, China in 2012 and worked as a Project Officer in School of Computer Science, Nanyang Technological University, Singapore 2012-2014. During her past PHD study, she has been dedicated to her research area of online learning for addressing the emerging challenges of big data analytics particularly for dealing with real-time data stream analytics. She has published several research papers as the first authors in top tier journals and high-impact conferences. Most of her research publications addressed the key open challenges in the area of machine learning and big data analytics fields.
|