|
Enriching Knowledge Graph Completion from Data and Model perspectives | 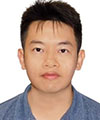 | LIU Ran PhD Candidate School of Computing and Information Systems Singapore Management University | Research Area Dissertation Committee Research Advisor Dissertation Committee Member |
| | Date 17 April 2024 (Wednesday) | Time 4:00pm – 5:00pm | Venue Meeting room 5.1, Level 5 School of Computing and Information Systems 1, Singapore Management University, 80 Stamford Road Singapore 178902 | Please register by 16 April 2024. We look forward to seeing you at this research seminar. 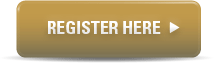
|
|
|
| About The Talk Knowledge graphs (KGs) have been widely used to encode facts about the real world. The rich facts contained in a large-scale knowledge graph can be used to enhance numerous applications that rely on real-world knowledge, such as question answering , object detection and recommendation. To effectively exploit the facts for various applications, a common approach is to first perform knowledge graph embedding (KGE) that converts the symbolic entities and relations to a latent vector space.
Although KGs are instrumental in various real-world applications, the performances of KGE models dramatically degrade in real-world scenarios due to the issue of data sparsity in knowledge graphs. In this dissertation proposal, we tackle the task of knowledge graph completion (KGC) and seek to address the issue of data sparsity from two different perspectives: (1) At the data level, we propose to improve the informativeness of negative triplets to compensate for the lack of positive triplets; (2) At the model level, we improve on few-shot relation learning (FSRL) models by adapting upstream meta-learned models to downstream relation tasks. Extensive experiments are conducted on benchmark datasets, which demonstrate the superiority of the proposed models. | | Speaker Biography Liu Ran is a PhD Candidate in Computer Science at the SMU School of Computing and Information Systems, supervised by Assistant Prof. Fang Yuan. His research is focused on Artificial Intelligence & Data Science and the main research interest lies in the topic of knowledge graph representation learning. |
|