|
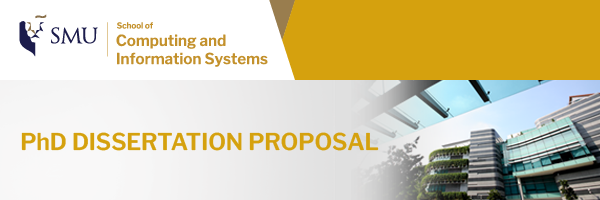 Continual Learning for Deep Models | 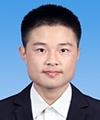 | LUO Zilin PhD Candidate School of Computing and Information Systems Singapore Management University | Research Area Dissertation Committee Research Advisor Committee Members |
| | Date 24 April 2025 (Thursday) | Time 3:30pm - 4:30pm | Venue Meeting room 5.1, Level 5 School of Computing and Information Systems 1, Singapore Management University, 80 Stamford Road Singapore 178902 | Please register by 23 April 2025. We look forward to seeing you at this research seminar. 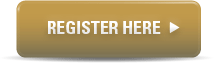
|
|
|
| ABOUT THE TALK Continual Learning (CL) is a deep learning task aimed at training models to continually learn new knowledge while preventing the forgetting of old knowledge. In this proposal, I will share two research works that discuss this problem from memory-based viewpoints. In the first work, the utilization of memory is considered by compressing exemplars through downsampling non-discriminative pixels, thereby preserving more semantic information. In the second work, the "phase" concept widely used in CL is reviewed and deemed unrealistic for two reasons: in-phase data stationarity and class balance in incoming data. To tackle the issues, per-step distribution shifts are introduced (thus eliminating phases) in the stream generation process. Under this setting, a coreset selector based on stream rates is proposed, presenting an adaptive and robust criterion. | | SPEAKER BIOGRAPHY Zilin LUO is a PhD Candidate in Computer Science at the SMU School of Computing and Information Systems, supervised by Prof. Qianru SUN. His research is focused on Continual Learning and In-Context Learning. |
|