|
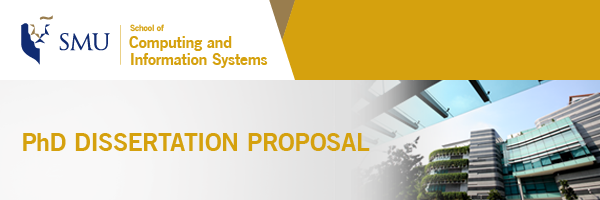 |
|
Using Explanation Methods in Sequential Recommendation
|
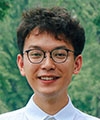
|
WANG Lei
PhD Candidate
School of Computing and Information Systems
Singapore Management University
|
Research Area
Dissertation Committee
Research Advisor
Committee Members
|
|
|
Date
24 November 2022 (Thursday)
|
Time
9:00am - 10:00am
|
Venue
Meeting room 5.1, Level 5
School of Computing and Information Systems 1,
Singapore Management University,
80 Stamford Road
Singapore 178902
|
Please register by 23 Nov 2022.
We look forward to seeing you at this research seminar.
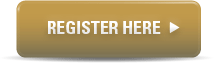
|
|
|
|
About The Talk
Recommender system is a critical component of today's online services to help users navigate the overwhelming amount of information and discover interesting items. In non-sequential recommendation models, items are recommended based on the user's general preferences without taking into account the order of user-time interactions. To model patterns embedded in the sequence of user-item interactions so as to make recommendation of the next item a user will interact with, many sequential recommendation models have been proposed. Recently, sequential recommendation models have achieved promising results through using neural networks to model user sequences. Nevertheless, there is still substantial room for sequential recommendation models to improve their accuracy and for them to generate results that can be explained to users. It turns out that both the above-mentioned accuracy and explainability challenges of sequential recommendation are closely related to explanation methods.
This report mainly covers two works that form my PhD research so far. Both works address the accuracy challenge in sequential recommendation using explanation methods. In the first work, We proposed an Explainable-guided Contrastive Learning for Sequential Recommendation (EC4SRec) framework to address the data sparsity problem and false positive and negative problems caused by contrastive learning-based sequential recommendation methods. First, EC4SRec utilizes explanation method(s) to determine items' importance in a user sequence and derives positive and negative sequences accordingly. Then, EC4SRec combines both self-supervised and supervised contrastive learning over the generated positive and negative sequences. In the second work, we propose Explanation-Guided Embedding Interpolation for Contrastive Learning-based Sequential Recommendation (E2ICL) model to address two fundamental pitfalls in existing augmentation strategies for contrastive learning-based sequential recommendation, namely, the information loss of the original anchor sequence (also known as the false positive problem) and the lack of flexibility and diversity of augmentations generated in a discrete item space. Specifically, E2ICL performs augmentation in the continuous embedding space by linear interpolation between representations of anchor sequences and their important items.
|
|
Speaker Biography
Lei Wang is a Ph.D. candidate at SMU SCIS, supervised by Prof. Lim Ee-Peng. His research focuses on improving sequential recommendation with explanation methods and developing explanation methods for the items suggested by sequential recommendation models.
|
|