|
Pre-Conference Talk for YU Sicheng,
TAN Minghuan and ZHENG Xiaosen
|
DATE : |
4 May 2022, Wednesday |
TIME : |
11.00am - 11.45am |
VENUE : |
This is a virtual seminar. Please register by 2 May, the zoom link will be sent out on the following day to those who registered. |
|
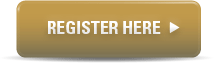
|
|
There are 3 talks in this session, each talk is approximately 15 minutes.
|
|
About the Talk (s)
Talk #1: Translate-Train Embracing Translationese Artifacts
by YU Sicheng, PhD Candidate
|
Translate-train is a general training approach to multilingual tasks. The key idea is to use the translator of the target language to generate training data to mitigate the gap between the source and target languages. However, its performance is often hampered by the artifacts in the translated texts (translationese). We discover that such artifacts have common patterns in different languages and can be modeled by deep learning, and subsequently propose an approach to conduct translate-train using Translationese Embracing the effect of Artifacts (TEA). TEA learns to mitigate such effect on the training data of a source language (whose original and translationese are both available), and applies the learned module to facilitate the inference on the target language. Extensive experiments on the multilingual QA dataset TyDiQA demonstrate that TEA outperforms strong baselines.
|
Talk #2: Exploring and Adapting Chinese GPT to Pinyin Input Method
by TAN Minghuan, PhD Candidate
|
In this work, we make the first exploration to leverage Chinese GPT for pinyin input method. We find that a frozen GPT achieves state-of-the-art performance on perfect pinyin. However, the performance drops dramatically when the input includes abbreviated pinyin. We mitigate this issue with two strategies, including enriching the context with pinyin and optimizing the training process to help distinguish homophones. To further facilitate the evaluation of pinyin input method, we create a dataset consisting of 270K instances from 15 domains. Results show that our approach improves the performance on abbreviated pinyin across all domains. Model analysis demonstrates that both strategies contribute to the performance boost.
|
Talk #3: An Empirical Study of Memorization in NLP
by ZHENG Xiaosen, PhD Candidate
|
A recent study by Feldman (2020) proposed a long-tail theory to explain the memorization behavior of deep learning models. However, memorization has not been empirically verified in the context of NLP, a gap addressed by this work. In this paper, we use three different NLP tasks to check if the long-tail theory holds. Our experiments demonstrate that top-ranked memorized training instances are likely atypical, and removing the top-memorized training instances leads to a more serious drop in test accuracy compared with removing training instances randomly.
|
These are pre-conference talks for 60th Annual Meeting of the Association for Computational Linguistics (ACL 2022).
About the Speaker(S)
|
|
|
|