|
Pre-Conference Talk for ZHANG Ce and TRAN Nhu Thuat
|
DATE : |
14 July 2022, Thursday |
TIME : |
10:30am - 12.00pm |
VENUE : |
This is a virtual seminar. Please register by 12 July, the zoom link will be sent out on the following day to those who registered. |
|
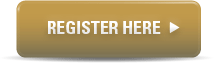
|
|
There are 3 talks in this session, each talk is approximately 30 minutes.
|
|
About the Talk (s)
Talk #1: Variational Graph Author Topic Modeling
by ZHANG Ce, PhD Candidate
|
While Variational Graph Auto-Encoder (VGAE) has presented promising ability to learn representations for documents, most existing VGAEs do not model a latent topic structure and lack semantic interpretability. Exploring hidden topics within documents and discovering key words associated with each topic allow us to develop a semantic interpretation of the corpus. Moreover, documents are usually associated with authors. Modeling authorship could benefit topic modeling, since documents by the same authors tend to reveal similar semantics. However, most topic models ignore the auxiliary authorship. Given above two challenges, we propose a Variational Graph Author Topic Model for documents to integrate both semantic interpretability and authorship modeling into a unified VGAE framework.
|
Talk #2: Aligning Dual Disentangled User Representations from Ratings and Textual Content
by TRAN Nhu Thuat, PhD Candidate
|
Classical recommendation methods typically render user representation as a single vector in latent space. Oftentimes, a user’s interactions with items are influenced by several hidden factors. To better uncover these hidden factors, we seek disentangled representations. Existing disentanglement methods for recommendations are mainly concerned with user-item interactions alone. To further improve not only the effectiveness of recommendations but also the interpretability of the representations, we propose to learn a second set of disentangled user representations from textual content and to align the two sets of representations with one another. Experiments conducted on four real-world datasets show the efficacy of our methods in improving recommendation quality.
|
Talk #3: Dynamic Topic Models for Temporal Document Networks
by ZHANG Ce, PhD Candidate
|
Dynamic topic models explore the time evolution of topics in temporally accumulative corpora. While existing topic models focus on the dynamics of individual documents, we propose two neural topic models aimed at learning unified topic distributions that incorporate both document dynamics and network structure. For the first model, by adding a time dimension, we propose Time-Aware Optimal Transport. For the second model, we further design a Temporal Point Process to capture the impact of historical neighbors on the current link formation. Experiments on four dynamic document networks demonstrate the advantage of our models.
|
For talk #1 and #2: These are pre-conference talks for The 28th ACM SIGKDD Conference on Knowledge Discovery and Data Mining (KDD 2022).
For talk #3: This is the pre-conference talk for The Thirty-Ninth International Conference on Machine Learning (ICML-22).
About the Speaker(S)
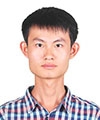 |
|
ZHANG Ce is a PhD Candidate in Computer Science at the SMU School of Computing and Information Systems, supervised by Prof. Hady W. Lauw. His research focuses on graph representation learning and text mining.
|
|
|
|
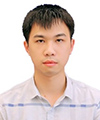 |
|
TRAN Nhu Thuat is a PhD Candidate in Computer Science at the SMU School of Computing and Information Systems, supervised by Prof. Hady W. Lauw. His central theme of research is interpretability in recommender systems.
|
|
|
|
|
|
|
|