|
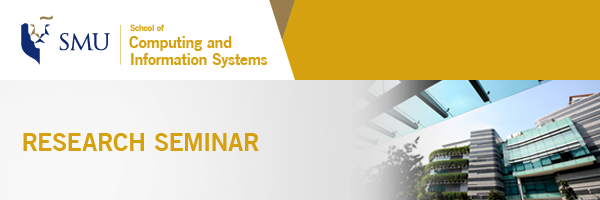 Machine learning for public health decision making Speaker (s): 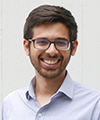 Bryan Wilder Assistant Professor, Carnegie Mellon University
| Date: Time: Venue: | | 3 April 2024, Wednesday 11:00am – 12:00pm School of Computing & Information Systems 1 (SCIS 1) Level 5, Meeting Room 5-1 Singapore Management University Singapore 178902 Please register by 30 March 2024. We look forward to seeing you at this research seminar. 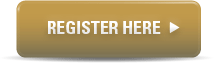
|
|
About the Talk Many policy settings, such as public health, require allocating limited resources under uncertainty about which individuals need those resources the most. Machine learning plays an increasing role in such allocations, most commonly to provide predictions of individual-level need. In this talk, I will discuss two recent works that take a more expansive view of the role of ML in resource allocation. First, I will discuss methods that allow a decision maker to optimally balance the immediate goal of targeting high-need individuals and the long-term goal of estimating treatment effects (i.e., does the program help people?). Our proposed method provides a policy for resource allocation which minimizes the variance with which the average treatment effect is estimated, subject to a constraint that at least some fraction of in-need individuals receive the intervention. Second, I will introduce ML as a lens to study the fairness of discretionary allocations by human decision makers, such as a clinician's decision to prescribe a resource-limited drug. Here the challenge is that human decisions are likely to be confounded (based on unobserved variables); we provide nontrivial bounds on allocative equity even in this challenging setting. In both works, we give both strong guarantees for the statistical and computational properties of our proposed methods as well as applications to real-world data from health and social service settings. About the Speaker Bryan Wilder is an Assistant Professor in the Machine Learning Department at Carnegie Mellon University. His research focuses on AI for equitable, data-driven decision making in high-stakes social settings, integrating methods from machine learning and optimization. Much of this work is motivated by public health applications, including maternal and child health, Covid-19, and HIV prevention. His research has been recognized with awards including a Schmidt AI2050 Early Career Fellowship, the IFAAMAS Victor Lesser Distinguished Dissertation Award, and best paper nominations at ICML and AAMAS.
|