|
Effort-Light StructMine: Turning Massive Text Corpora into Structures
Speaker (s):
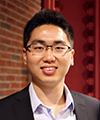
Xiang Ren
Visiting Researcher
Stanford University
|
Date:
Time:
Venue:
|
|
November 9, 2017, Thursday
3:00pm - 4:00pm
Meeting Room 5.1, Level 5
School of Information Systems
Singapore Management University
80 Stamford Road
Singapore 178902
We look forward to seeing you at this research seminar.
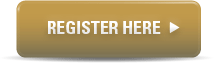
|
|
ABSTRACT
The real-world data, though massive, are hard for machines to resolve as they are largely unstructured and in the form of natural-language text. One of the grand challenges is to turn such massive corpora into machine-actionable structures. Yet, most existing systems have heavy reliance on human effort in the process of structuring various corpora, slowing down the development of downstream applications.
In this talk, I will introduce a data-driven framework, Effort-Light StructMine, that extracts structured facts from massive corpora without explicit human labeling effort. In particular, I will discuss how to solve three structure mining tasks under Effort-Light StructMine framework: from identifying typed entities in text, to fine-grained entity typing, to extracting typed relationships between entities. Together, these three solutions form a clear roadmap for turning a massive corpus into a structured network to represent its factual knowledge. Finally, I will share some directions towards mining corpus-specific structured networks for knowledge discovery.
About the Speaker
Xiang Ren is a visiting researcher at Stanford University and an incoming Assistant Professor of Computer Science at USC. He will defend his PhD in Computer Science at University of Illinois at Urbana-Champaign later this year, where he was a Richard T. Cheng Fellow working with Prof. Jiawei Han. His research focuses on developing automated and scalable techniques for turning massive text data into machine-actionable structures and applying structured knowledge to power intelligent services. He is particularly interested in designing effective computational models for partialy- and noisily-labeled data, learning with complex label space, automating knowledge base construction, and knowledge acuquistion with human in the loop. Xiang's research has been recognized with several prestigious awards including a Google PhD Fellowship, a Yahoo!-DAIS Research Excellence Award, a Yelp Dataset Challenge award, a C. W. Gear Outstanding Graduate Student Award and a David J. Kuck Outstanding M.S. Thesis Award. Technologies he developed has been transferred to US Army Research Lab, National Institute of Health, Microsoft, Yelp and TripAdvisor.
|