|
Research Seminar by ZHU Jiawen | DATE : | 14 June 2024, Friday | TIME : | 3:00pm to 3:45pm | VENUE : | Meeting room 4.4, Level 4 School of Computing and Information Systems 1, Singapore Management University, 80 Stamford Road, Singapore 178902
Please register by 23 October 2024 |
| 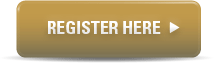
|
* There are 2 talks in this session, each talk is approximately 25 minutes.* All sessions are for pre-conference talk for IEEE / CVF Computer Vision and Pattern Recognition Conference (CVPR 2024). | About the Talk (s) Talk #1: Anomaly Heterogeneity Learning for Open-set Supervised Anomaly Detection | Open-set supervised anomaly detection (OSAD) – a recently emerging anomaly detection area – aims at utilizing a few samples of anomaly classes seen during training to detect unseen anomalies (i.e., samples from open-set anomaly classes), while effectively identifying the seen anomalies. Benefiting from the prior knowledge illustrated by the seen anomalies, current OSAD methods can often largely reduce false positive errors. However, these methods are trained in a closed-set setting and treat the anomaly examples as from a homogeneous distribution, rendering them less effective in generalizing to unseen anomalies that can be drawn from any distribution. This paper proposes to learn heterogeneous anomaly distributions using the limited anomaly examples to address this issue. To this end, we introduce a novel approach, namely Anomaly Heterogeneity Learning (AHL), that simulates a diverse set of heterogeneous anomaly distributions and then utilizes them to learn a unified heterogeneous abnormality model in surrogate open-set environments. Further, AHL is a generic framework that existing OSAD models can plug and play for enhancing their abnormality modeling. Extensive experiments on nine real-world anomaly detection datasets show that AHL can 1) substantially enhance different state-of-the-art OSAD models in detecting seen and unseen anomalies, and 2) effectively generalize to unseen anomalies in new domains. | Talk #2: Toward Generalist Anomaly Detection via In-context Residual Learning with Few-shot Normal Sample Prompts | This paper explores the problem of Generalist Anomaly Detection (GAD), aiming to train one single detection model that can generalize to detect anomalies in diverse datasets from different application domains without any further training on the target data. Some recent studies have shown that large pre-trained Visual-Language Models (VLMs) like CLIP have strong generalization capabilities on detecting industrial defects from various datasets, but their methods rely heavily on handcrafted text prompts about defects, making them difficult to generalize to anomalies in other applications, e.g., medical image anomalies or semantic anomalies in natural images. In this work, we propose to train a GAD model with few-shot normal images as sample prompts for AD on diverse datasets on the fly. To this end, we introduce a novel approach that learns an in-context residual learning model for GAD, termed InCTRL. It is trained on an auxiliary dataset to discriminate anomalies from normal samples based on a holistic evaluation of the residuals between query images and few-shot normal sample prompts. Regardless of the datasets, per definition of anomaly, larger residuals are expected for anomalies than normal samples, thereby enabling InCTRL to generalize across different domains without further training. Comprehensive experiments on nine AD datasets are performed to establish a GAD benchmark that encapsulate the detection of industrial defect anomalies, medical anomalies, and semantic anomalies in both one-vsall and multi-class setting, on which InCTRL is the best performer and significantly outperforms state-of-the-art competing methods. |
| | | About the Speaker 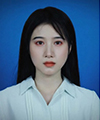 | | Zhu Jiawen is a PhD Candidate supervised by Prof. Pang Guansong. Her research interests are Anomaly Detection. |
|
|
|